DataIA Seminar | Diffusion models and Representation Learning in Computer Vision
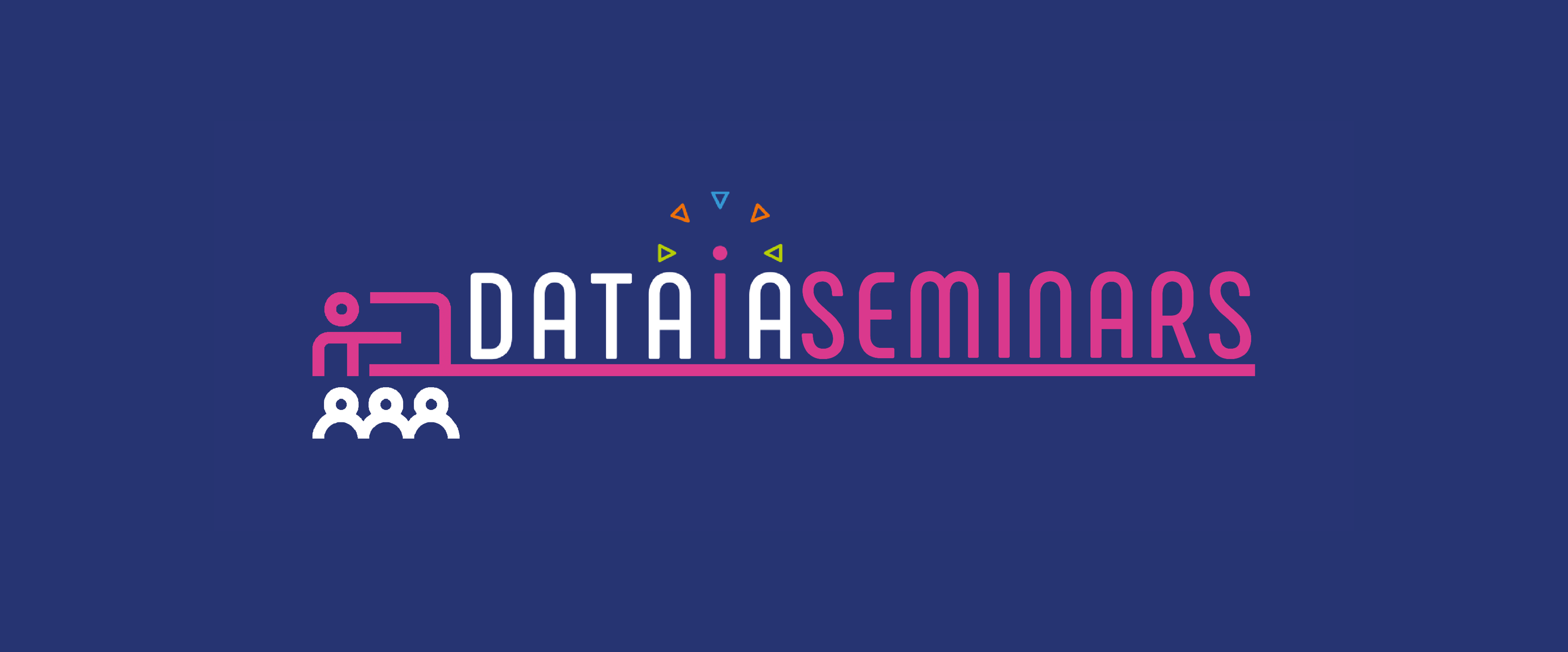
Meet us at Amphitheater I of the Eiffel Building at CentraleSupélec, from 1 to 2 pm!
Title - Speakers
Diffusion models and Representation Learning in Computer Vision
Srikar Yellapragada, Varun Belagali and Minh Quan Le
Abstract
Diffusion models are powerful methods for image generation that are commonly used in the computer vision community for a variety of applications, including self-supervised learning and video generation. In this talk, we will highlight four different works of our team framed around diffusion processes. In particular, we will introduce ZoomLDM [1], a multi-scale diffusion model enabling high-quality synthesis of gigapixel-scale imagery. We will also talked about Gen-SIS [2], a novel diffusion-based augmentation technique for self-supervised learning that operates solely on unlabeled data. Furthermore, we will present Hummingbird [3], the first diffusion model designed for scene-aware tasks, achieving high fidelity in preserving multimodal context while maintaining generation diversity. Finally, we will also present ODDISH [4], Optimal Transport-based Alignment Rewards, which improves the quality and semantic alignment of text-to-video generation.
A part of the talk will present as well our current work in the frame of DataIA and in particular, the collaborations we have established through our visit in CentraleSupelec. We will talk about CDG-MAE, a novel pre-training strategy that leverages the diversity of synthetic images generated by diffusion models, incorporating a multi-masking technique. This method significantly improves performance on challenging view correspondence tasks such as video object propagation, semantic part propagation, and pose propagation, outperforming existing state-of-the-art without requiring video data for pre-training. Moreover, for video generation, to improve the physical laws in pre-trained video diffusion models, we will present a novel multi-agent framework for scalable and automatic simulation of physical properties from text prompt and incorporate them into the video generation process. We also come up with a post-training approach which employs Physics MLLMs to evaluate the physical plausibility of generated videos and uses that reward as feedback to improve physical laws in pre-trained video diffusion models.
[1] Yellapragada et al. ZoomLDM: Latent Diffusion Model for multi-scale image generation. CVPR 2025
[2] Belagali et al. Gen-SIS: Generative Self-augmentation Improves Self-supervised Learning. arXiv 2024
[3] Le et al. Hummingbird: High Fidelity Image Generation via Multimodal Context Alignment. ICLR 2025
[4] Le et al. ODDISH: Optimal Transport-based Alignment Rewards for Enhanced Text-to-Video Generation. Arxiv 2025