« Le Séminaire Palaisien » | Ulugbek Kamilov & Arshak Minasyan
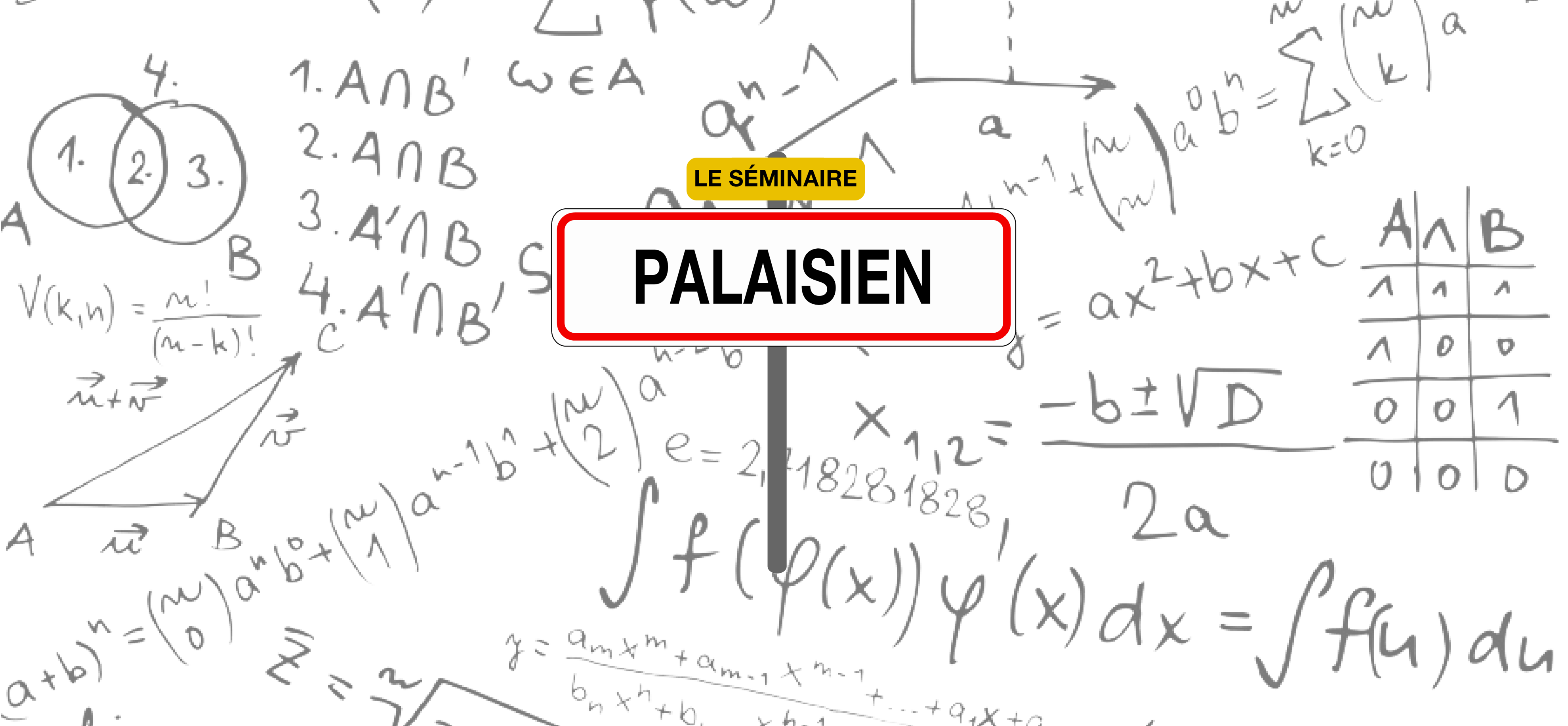
Each seminar session is divided into two scientific presentations of 40 minutes each: 30 minutes of talk and 10 minutes of questions.
Ulugbek Kamilov and Arshak Minasyan will host the April 2024 session!
Registration is free but compulsory, subject to availability. A buffet will be served at the end of the seminar.
Abstract
We propose two estimators for estimating the unknown mean and covariance matrix of anisotropic Gaussians that achieve the minimax optimal rate in the presence of outliers. The contamination model considered is the adversarial/strong contamination model, which contains all well-known contamination models, e.g., Huber's contamination model. Despite the recent significant interest in robust statistics, achieving both dimension-free bounds in the canonical Gaussian case remained open. In fact, several previously known results were either dimension-dependent and required the covariance matrix to be close to identity or had a sub-optimal dependence on the contamination level.
As a part of the analysis, we derive sharp concentration inequalities for central order statistics of Gaussian, folded normal, and chi-squared distributions. This is a joint work with N. Zhivotovskiy.
Abstract
Many interesting computational imaging problems can be formulated as imaging inverse problems. Since these problems are often ill-posed, one needs to integrate all the available prior knowledge for obtaining high-quality solutions. This talk focuses on the class of methods based on using “image restoration” deep neural network as data-driven implicit priors on images. The roots of the methods discussed in this talk can be traced to the popular Plug-and-Play Priors (PnP) family of methods for solving inverse problems. The talk will present applications of learned implicit priors for image generation in limited angle computed tomography, recovery of continuously represented microscopy images, and solving blind inverse problems in magnetic resonance imaging.