Workshop | "Mathematical Foundations of AI"
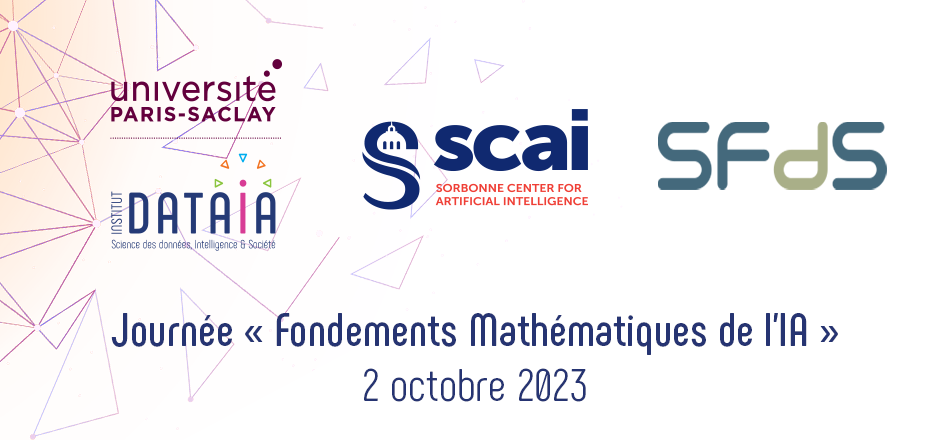
The "Mathematical Foundations of AI" workhop, organized jointly by the DATAIA Institute, SCAI and the MALIA Group of the French Statistical Society aims to provide an overview of some promising research directions at the interface between statistical learning and AI. The mathematics of deep learning (approximation theory, stability/robustness analysis) and optimization for learning, or generative models will all be addressed during the day.
The morning will be devoted to plenary talks by world-renowned researchers, while the afternoon will be an opportunity for young researchers to present their work via posters or short talks.
Organizing Committee
- Marianne Clausel (Lorraine University)
- Emilie Chouzenoux (INRIA Saclay, DATAIA Institute)
- MALIA Group of the French Statistical Society
Scientific Committee
- Stéphan Chrétien (Lyon 2 University)
- Sylvain Le Corff (Sorbonne University)
- Konstantin Usevich (CNRS)
- Myriam Tami (CentraleSupélec)
Workshop room : 44-54 tower, room 107
Break room (coffee, lunch) : 2400 tower (Zamansky)
- 9am-10am | Keynote I : Arnaud Doucet (Oxford University & Google DeepMind)
"From Denoising Diffusion Models to Dynamic Transport Models - Generative Modeling and Inference"
Denoising diffusion models are a powerful new class of techniques for generative modeling and inference. These models have supplanted generative adversarial networks in the last two years, as they are flexible, easy to train and provide state-of-the-art results in many application areas such as image synthesis and protein design. They are, for example, at the heart of popular text-to-image models such as Dalle2, Imagen, Midjourney and Stable Diffusion. In this talk, we will review these methods, illustrate them on a variety of applications and discuss their limitations. We will then show how recent alternative techniques based on dynamic mass transport ideas can resolve some of these limitations. In particular, we will focus on Schrödinger bridges, an entropy-regularized version of dynamical optimal transport, and present a simple new method for approaching them numerically.
Biography
Arnaud Doucet obtained his PhD in Information Engineering from the University of Paris-XI in 1997. Since then, he has held professorial positions at the University of Cambridge, the University of Melbourne, the University of British Columbia and the Statistical Mathematics Institute in Tokyo. He joined the Department of Statistics at Oxford University in 2011, where he is currently Statutory Professor (Oxford Speaks for the Chair). Since 2019, he has also been a Senior Researcher at Google DeepMind. He was Medal Lecturer of the Institute of Mathematical Statistics (IMS) in 2016, was elected Fellow of the IMS in 2017 and was awarded the Guy Silver Medal of the Royal Statistical Society in 2020.
- 10am-10:40am | Session 1 : 2 contributed talks
Ségolène Martin (CVN, CentraleSupélec) | "Adressing class unbalance in transductive few-shot learning"
In this talk, we will explore the challenges and limitations of existing few-shot learning benchmarks and introduce a more flexible and realistic approach. Traditional benchmarks often rely on assumptions that don’t always align with real-world scenarios, such as class balance, limiting their effectiveness. Addressing this, we present a new formulation, the PrimAl Dual Minimum Description LEngth (PADDLE), which offers a composite optimization-based approach to handling data accuracy and model complexity. This method fosters competition among a vast range of possible classes, ensuring only the most relevant are retained for a task. Notably, PADDLE is hyperparameter-free and highly adaptable to various training bases. We will also discuss a developed algorithm for minimizing the loss function, which guarantees convergence and offers computational efficiency. Finally, comprehensive experiments demonstrate the effectiveness of the method.
Raphaël Mignot (Université de Lorraine) | "Averaging time series, a new approach with the signature method"
The aim of our work is to average multidimensional time series. We encode time series with integrals of various moment orders, constituting their signature. We compute the mean in this feature space, which has a Lie group structure. This allows us to leverage the beneficial properties of signatures for ubiquitous machine learning tasks: clustering, data augmentation, dimension reduction.
- 10:40am-10:55am | Coffee break
- 11am-12pm | Keynote II : Cordelia Schmidt (INRIA)
Title
"Multimodal video representations and their extension to visual language navigation"
Abstract
In this talk, we present recent advances in large-scale learning of multimodal video representations. We begin by presenting VideoBert, a joint model for video and language, which adapts the Bert model to multimodal data. This model provides state-of-the-art results for zero-beat prediction and video captioning. Next, we present Vid2Seq, a dense video captioning model that takes video and speech as input and simultaneously predicts temporal boundaries and textual descriptions. We then present an approach to video question answering and image captioning based on a retrieval-augmented visual language model that learns to encode knowledge of the world in large-scale memory and retrieve it to answer knowledge-intensive queries. We show that our approach achieves state-of-the-art results in visual query answering and image captioning. We conclude the presentation with recent work on vision-guided navigation and robot manipulation based on linguistic instructions. This work builds on and extends vision-language transformers by integrating action history and action prediction. We outperformed the state-of-the-art on various vision- and language-guided navigation testbeds and on RLBench, a robot manipulation testbed.
Biography
Cordelia Schmidt holds an MSc in Computer Science from the University of Karlsruhe and a PhD in Computer Science from the Institut National Polytechnique de Grenoble (INPG). Her doctoral thesis, entitled "Local Greyvalue Invariants for Image Matching and Retrieval", was awarded the INPG prize for the best thesis in 1996. She was awarded the diploma of habilitation in 2001 for her thesis entitled "From Image Matching to Learning Visual Models". Dr. Schmidt was a post-doctoral research assistant in the Robotics Research Group at Oxford University in 1996-1997. Since 1997, she has held a permanent research position at Inria, where she is Research Director. Dr. Schmidt is a member of the German National Academy of Sciences, the Leopoldina, the IEEE and the ELLIS Society. She was awarded the Longuet-Higgins Prize in 2006, 2014 and 2016 and the Koenderink Prize in 2018, both for fundamental contributions to computer vision that have stood the test of time. She was awarded an ERC Advanced Fellowship in 2013, the Humbolt Research Prize in 2015, the Grand Prix Inria & Académie des sciences de France in 2016, the Royal Society Milner Prize in 2020, the PAMI distinguished researcher award in 2021 and the Körber European Science Price in 2023. Ms. Schmid was Associate Editor of IEEE PAMI (2001--2005) and IJCV (2004--2012), Editor-in-Chief of IJCV (2013--2018), Program Chair of IEEE CVPR 2005 and ECCV 2012, and General Chair of IEEE CVPR 2015, ECCV 2020 and ICCV 2023. Since 2018, she has held a joint position with Google Research.
- 12:15pm-1:45pm | Lunch break
- 1:45pm-2:45pm | Keynote III : Rémi Flamary (CMAP)
Title
"Optimal Transport for Machine Learning: 10 years of least effort"
Abstract
Optimal transport (OT) has become an important tool in the machine learning community in recent years. This has been made possible by new formulations and optimization algorithms such as the Sinkhorn distance, proposed 10 years ago. In this presentation, we will give a brief introduction to numerical optimal transport and its optimization algorithms. We will then discuss two important aspects of optimal transport, namely the Wasserstein distance and the OT correspondence between distributions. Finally, we will present the different ways in which numerical optimal transport has been used in machine learning applications, both supervised and unsupervised learning methods.
Biography
Remi Flamary is a professor at École Polytechnique's Centre de Mathématiques Appliquées (CMAP) and holds a 3IA Côte d'Azur Chair in Artificial Intelligence. He was previously an MCF at the Université Côte d'Azur (UCA) and a member of the Lagrange Laboratory at the Observatoire de la Côte d'Azur. He obtained an engineering degree in electrical engineering and a master's degree in image processing from the Institut National de Sciences Appliquées de Lyon in 2008, a doctorate from the Université de Rouen in 2011, and a habilitation to direct research (HDR) from the Université Côte d'Azur in 2019. His current research interests include signal and image processing, and machine learning, with a recent focus on applications of optimal transport theory to machine learning problems such as graph processing and domain adaptation.
- 2:50pm-3:50pm | Keynote IV : Liva Ralaivola (Criteo)
- 4pm-4:15pm | Coffee break
- 4:20pm-5:20pm | Session 2 : 2 contributed talks
Nathan Buskulic (GREYC, Caen University) | "Convergence guarantees for unsupervised neural networks applied to inverse problems"
In recent years, neural networks have become a major tool for solving inverse problems. However, the theoretical understanding of these models remains rather incomplete. This presentation will show theoretical convergence guarantees for these gradient-flow trained networks. We will first discuss a first result indicating that for any cost function respecting the Kurdyka-Lojasiewicz inequality, training a network under certain conditions on its Jacobian will produce a zero-cost solution. We will then present a second result allowing us to obtain deterministic guarantees on the reconstruction of the underlying signal under a condition of restricted injectivity of the operator. We will then show how to use the notion of overparametrization on a two-layer network to control the Jacobian of the network in the desired way and thus obtain with high probability the guarantees discussed earlier.
El Mehdi Achour (RWTH Aachen University) | "The loss landscape of deep linear networks: A second-order analysis"
We study the empirical risk optimization landscape of deep linear neural networks with least-squares loss. It is known that, under weak assumptions, there are no local non-global minimizers and no local maximizers. However, the existence and diversity of non-strict saddle points, which may play a role in the dynamics of first-order algorithms, has been little studied. We provide a comprehensive analysis of the second-order optimization landscape, characterizing, among all critical points, global minimizers, strict saddle points and non-strict saddle points. We list all associated critical values. The characterization is simple, involves conditions on the ranks of matrix partial products, and sheds light on the global convergence or implicit regularization that have been proven or observed in the optimization of linear neural networks. In passing, we provide an explicit parameterization of the set of all global minimizers and expose large sets of strict and non-strict saddle points.