Le Séminaire Palaisien | Machine Learning and Statistics
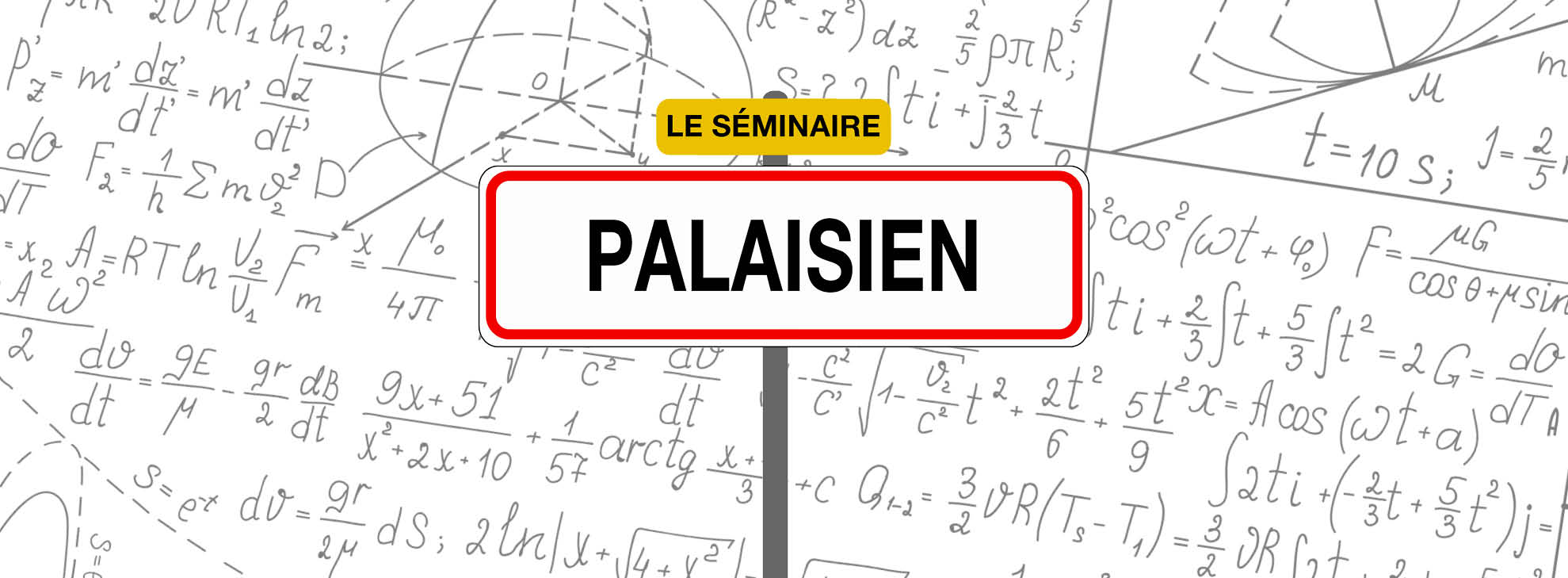
Each seminar session is divided into 2 scientific presentations of 40 minutes each: 30 minutes of presentation and 10 minutes of questions, followed by a coffee break.
Imke Mayer and Vianney Perchet, will lead the session of January 7th.
Inferring causal effects of a treatment or policy from observational data is central to many applications. However, state-of-the-art methods for causal inference seldom consider the possibility that covariates have missing values, which is ubiquitous in many real-world cases.
This work is motivated by assessing several medical questions about different treatments based on a large prospective database counting over 20,000 major trauma patients. This database is complex in the sense that it presents a multi-level and heterogeneous structure and precisely contains large fractions of missing values.
Missing data greatly complicate causal analyses as they either require strong assumptions about the missing data generating mechanism or an adapted unconfoundedness hypothesis. In this talk, I will first provide a classification of existing methods according to the main underlying assumptions, which are based either on variants of the classical unconfoundedness assumption or relying on assumptions about the mechanism that generates the missing values. Then, I will present two recent contributions on this topic: (1) an extension of doubly robust estimators that allows handling of missing attributes, and (2) an approach to causal inference based on variational autoencoders adapted to incomplete data.
Information is valuable either by remaining private (for instance if it is sensitive) or, on the other hand, by being used publicly to optimize some target loss functions. These two objectives are antagonistic and leaking this information might be more rewarding than concealing it. Unlike classical solutions that focus on the first point, we consider instead agents that maximize a natural trade-off between both objectives.
We will in a first step quickly review some concepts of privacy in machine learning, before formalizing the tradeoff utility vs privacy as an optimization problem where the objective mapping is regularized by the amount of information revealed to the adversary (measured as a divergence between the prior and posterior on the private knowledge).
Quite surprisingly, when combined with the entropic regularization, the Sinkhorn loss naturally emerges in the optimization objective, making it efficiently solvable. We apply these techniques to preserve some privacy in online repeated auctions.
The seminar will be followed by a coffee break.
Registration free but mandatory within the limit of available seats.
For security reasons, no access to the conference room for unregistered participants