Séminaire DATAIA | Enzo Ferrante « Improving anatomical plausibility and auditing fairness in deep segmentation networks »
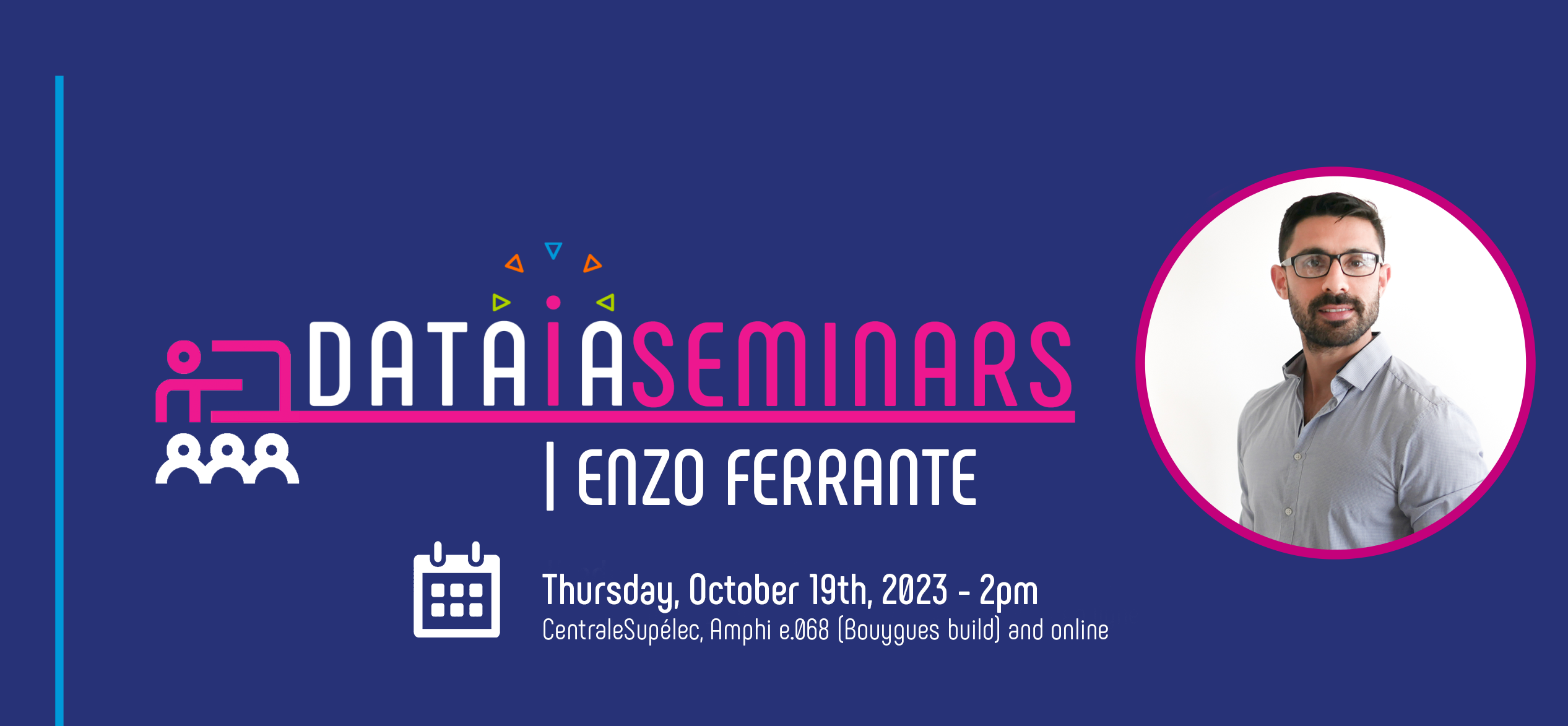
Retrouvez dès maintenant le replay du séminaire et la présentation de l'orateur !
Titre
Improving anatomical plausibility and auditing fairness in deep segmentation networks
Résumé
The evolution of deep segmentation networks has empowered the enhancement of extensive medical imaging datasets with automatically generated anatomical segmentation masks. In this talk we will discuss recent methods we proposed to improve anatomical plausibility in deep segmentation networks. By improving anatomical plausibility we mean to ensure that the segmentation masks produced by our network are constrained to the actual shape and appearance of organs. We will briefly discuss some of our studies [1,2,3] which use autoencoders to learn low dimensional embeddings of anatomical structures and propose different ways in which they can be incorporated into deep learning models for segmentation and registration.
The complexity is further intensified by recent studies indicating potential biases in AI-based medical imaging models related to gender, age, and ethnicity [4,5]. Here we will share insights from our journey in developing the CheXMask large-scale database of x-ray anatomical segmentations [6]. We will delve into the strategies we implemented for automatic quality control and the methods we formulated for unsupervised bias discovery in the absence of ground-truth annotations [7].
[1] Learning deformable registration of medical images with anatomical constraints | Mansilla L, Milone D, Ferrante E.
Neural Networks (2020)
[2] Post-DAE: Anatomically Plausible Segmentation via Post-Processing with Denoising Autoencoders | Larrazabal A, Martinez C, Glocker B, Ferrante E.
IEEE Transactions on Medical Imaging (2020)
MICCAI 2019 (conference version)
[3] HybridGNet - Improving anatomical plausibility in image segmentation via hybrid graph neural networks: applications to chest x-ray image analysis | Gaggion N, Mansilla L, Mosquera C, Milone D, Ferrante E.
IEEE Transactions on Medical Imaging (2022)
MICCAI 2021 (conference version)
[4] Gender imbalance in medical imaging datasets produces biased classifiers for computer-aided diagnosis | Larrazabal AJ, Nieto N, Peterson V, Milone DH, Ferrante E.
Proceedings of the National Academy of Sciences (2020)
[5] Addressing fairness in artificial intelligence for medical imaging | Ricci Lara MA, Echeveste R, Ferrante E.
Nature communications (2022)
[6] CheXmask: a large-scale dataset of anatomical segmentation masks for multi-center chest x-ray images | Gaggion N, Mosquera C, Mansilla L, Aineseder M, Milone DH, Ferrante E.
arXiv preprint (2023)
[7] Unsupervised bias discovery in medical image segmentation | Gaggion N, Echeveste R, Mansilla L, Milone DH, Ferrante E.
MICCAI FAIMI Workshop (2023)
Biographie
Dr. Enzo Ferrante a obtenu son doctorat en informatique à l'Université Paris-Saclay et à l'INRIA (Paris, France), et a travaillé comme chercheur postdoctoral à l'Imperial College London au Royaume-Uni. Il a également été étudiant invité au CVGL Lab de l'Université de Stanford (2014), chercheur invité Fulbright au A. Martinos Center for Biomedical Imaging (Massachusetts General Hospital - Harvard Medical School, 2021) à Boston, et occupe actuellement un poste de professeur invité DATAIA au MICS Lab, CentraleSupélec, Université Paris-Saclay. Il est retourné en Argentine en 2017, où il occupe un poste de chercheur au Conseil National de la Recherche d'Argentine (CONICET). Il est régulièrement membre du comité de programme d'importantes conférences sur l'imagerie médicale telles que MICCAI, MIDL, ISBI et IPMI, et il a organisé plusieurs ateliers dans ce contexte
Il dirige la ligne de recherche Machine Learning for Biomedical Image Computing au sein de l'Institut de recherche sur les signaux, les systèmes et l'intelligence computationnelle, sinc(i). En 2020, le Dr Ferrante a reçu le prix du jeune chercheur de l'Académie nationale des sciences d'Argentine et le prix Mercosur de la science et de la technologie pour ses contributions scientifiques à l'intelligence artificielle pour l'informatique des images médicales. Ses recherches portent sur l'intelligence artificielle et l'analyse d'images biomédicales, et se concentrent actuellement sur l'équité, l'adaptation et la généralisation des domaines, l'étalonnage et la segmentation anatomique des images médicales.
- Le séminaire, co-organisé avec le laboratoire MICS de CentraleSupélec, se tiendra en anglais uniquement. Il aura lieu le jeudi 19 octobre 2023, de 14h à 15h30 à CentraleSupélec, Amphi e.068 (bâtiment Bouygues). Il sera suivi d'une pause sucrée.
- Ce séminaire sera également retransmis en visioconférence.
Ne ratez pas l'annonce d'un nouveau séminaire DATAIA !
Inscrivez-vous à la liste de diffusion de nos séminaires en cliquant ici.