« Le Séminaire Palaisien » | Alejandro de la Concha & Xavier d’Haultfoeuille
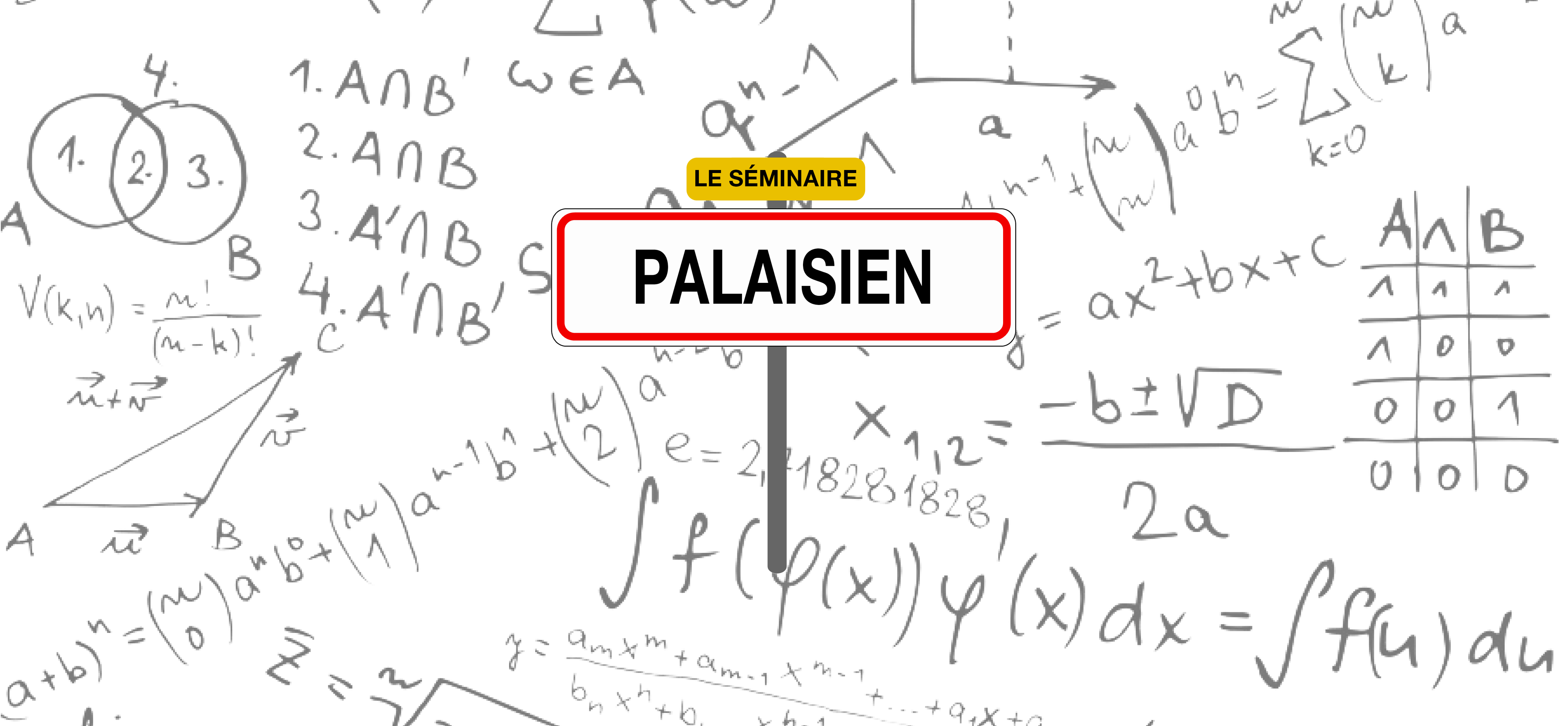
Chaque session du séminaire est divisée en deux présentations scientifiques de 40 minutes chacune : 30 minutes d’exposé et 10 minutes de questions.
Alejandro de la Concha et Xavier d’Haultfoeuille animeront la session de juin 2023.
Inscriptions gratuites mais obligatoires, dans la limite des places disponibles. Un buffet sera servi à l'issue du séminaire.
Résumé : assuming we have i.i.d observations from two unknown probability density functions (pdfs), p and p′, the likelihood-ratio estimation (LRE) is an approach to compare two pdfs without knowing their functional form explicitly. In this talk, we will introduce a graph-based extension of the problem. We will suppose each node v of a fixed graph has access to observations coming from two unknown node-specific pdfs, p_v and p′_v; the goal is then to compare the respective p_v and p′_v of each node by integrating information provided by the graph structure. This setting is interesting when the graph conveys some sort of 'similarity' between the node-wise estimation tasks, which suggests that the nodes can collaborate to solve more efficiently their individual tasks. Our main contribution is a distributed non-parametric framework for graph-based LRE, called GRULSIF, that incorporates in a novel way elements from f-divergence functionals, Kernel methods, and Multitask Learning. Among the applications of LRE, we choose the two-sample hypothesis testing to develop a proof of concept for our graph-based learning framework. Our experiments compare favorably the performance of our approach against state-of-the-art non-parametric statistical tests that apply at each node independently, and thus disregard the graph structure.
Résumé : we study partially linear models when the outcome of interest and some of the covariates are observed in two different datasets that cannot be linked. This type of data combination problem arises very frequently in empirical microeconomics. Using recent tools from optimal transport theory, we derive a constructive characterization of the sharp identified set. We then build on this result and develop a novel inference method that exploits the specific geometric properties of the identified set. Our method exhibits good performances in finite samples, while remaining very tractable. We apply our approach to study intergenerational income mobility over the period 1850-1930 in the United States. Our method allows us to relax the exclusion restrictions used in earlier work, while delivering confidence regions that are informative.