SEMINAIRE | Distributionally Robust Learning – From Traditional to Deep and to Reinforcement Learning
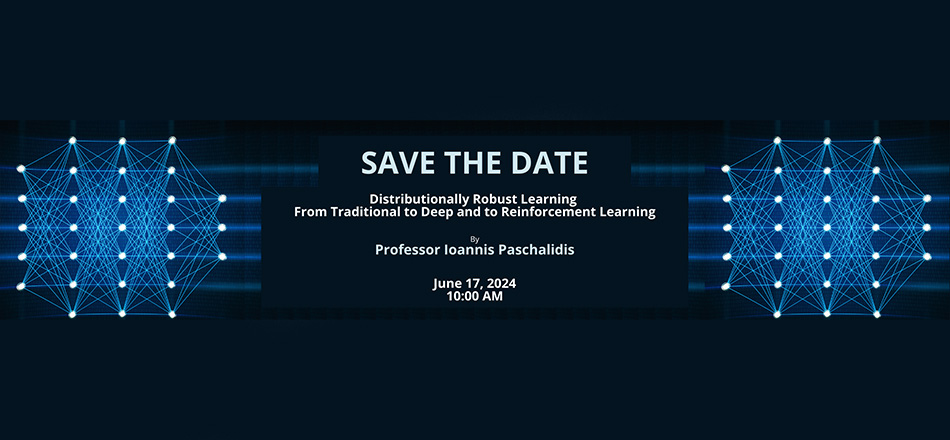
Résumé
In this talk I will present a distributionally robust optimization approach to machine learning, using general loss functions that can be used either in the context of classification or regression. Motivated by medical applications, we consider a setting where training data may be contaminated with (unknown) outliers. The robust learning problem is formulated as the problem of minimizing the worst case expected loss over a family of distributions that are close to the empirical distribution obtained from the training data. We will explore the generality of this approach, its robustness properties, its ability to explain a host of "ad-hoc" regularized learning methods, and we will establish rigorous out-of-sample performance guarantees. Beyond predictions, we will discuss methods that can leverage the robust predictive models to make decisions and offer specific personalized prescriptions and recommendations to improve future outcomes. We will also discuss how distributionally robust learning can be used in deep neural network classification, considering applications in computer vision. Finally, we will discuss how this framework can be used for safe reinforcement learning, solving a robust variant of a constrained Markov Decision Process problem with applications in robotics and autonomous systems.