« Le Séminaire Palaisien » | Tony Silveti-Falls & Erwan Allys
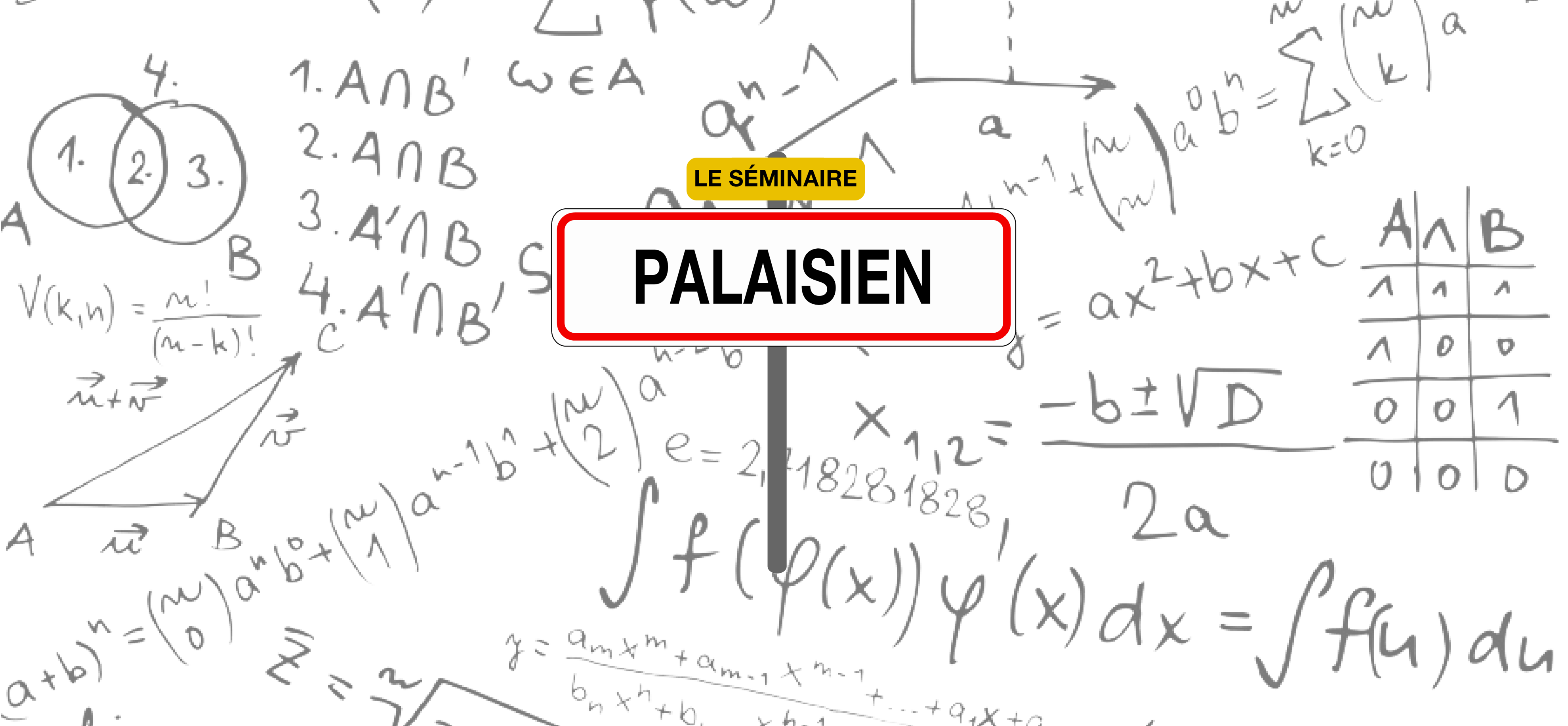
Each seminar session is divided into two scientific presentations of 40 minutes each: 30 minutes of talk and 10 minutes of questions. Tony Silveti-Falls & Erwan Allys will host the May 2025 session!
Registration is free but compulsory, subject to availability. A buffet will be served at the end of the seminar.
Abstract
In this talk, I discuss optimization methods that leverage the linear minimization oracle (LMO) over a norm-ball and their application to training huge neural networks. We propose a new stochastic family of algorithms that uses the LMO to adapt to the geometry of the problem and, perhaps surprisingly, show that they can be applied to unconstrained problems. The resulting update rule unifies several existing optimization methods under a single framework. Furthermore, we propose an explicit choice of norm for deep architectures, which, as a side benefit, leads to the transferability of hyperparameters across model sizes. Experimentally, we demonstrate significant speedups on nanoGPT training without any reliance on Adam. The proposed method is memory-efficient, requiring only one set of model weights and one set of gradients, which can be stored in half-precision.
Abstract
Scattering transform statistics have led to recent advances in the modelling of physical processes. These statistics, which are inspired by neural networks but can be estimated without a training step, allow quantitative modelling of physical processes, in a maximum entropy framework, even from very small data sets. After introducing these models and quantitatively demonstrating their quality on several examples, I will discuss how they can form the basis of new algorithms for inverse problems and component separation. In particular, I will show how they can be used to separate components even in a very limited data regime and without physically-driven priors of the component of interest, with examples of applications to astrophysical data.