StreamOps: a robust, performing and easily scalable streaming platform
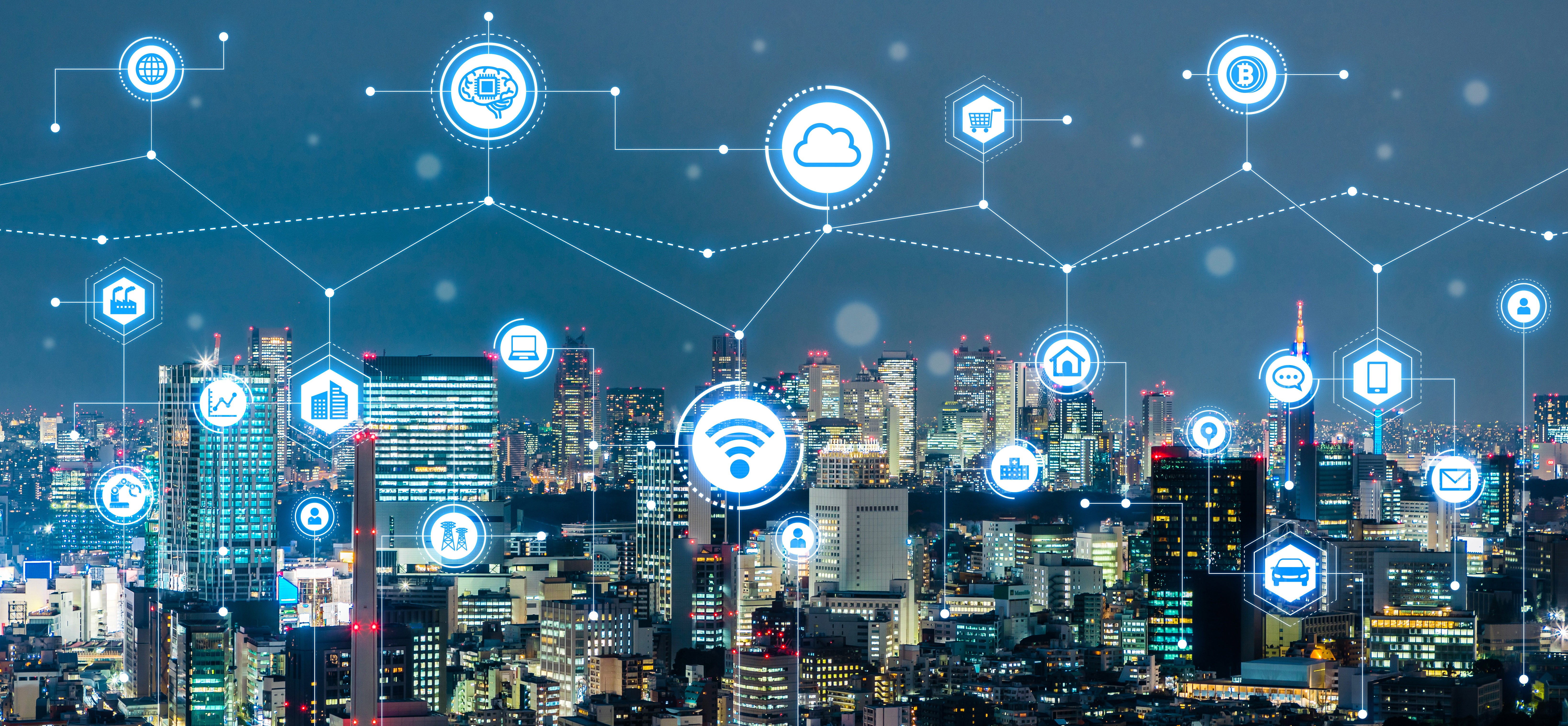
StreamOps: a robust, performing and easily scalable streaming platform
- News on the same topic
A generic but cutting-edge streaming platform
The scientific community develops algorithms to manage data flows. The industrials want to analyze the data for their use in applications. In computing, extremely powerful tools in terms of data and robustness are developed. "With the StreamOps project, we want to position ourselves at the interface of algorithmic aspects, business and software to offer all stakeholders a generic streaming platform but at the forefront of algorithms", explains Cédric.
Indeed, the ambition of StreamOps is to simultaneously meet the following objectives:
- performance in detection (reactivity, accuracy), performance in compression of information, confidentiality of data
- problems related to real data
- easy integration of new algorithms
- operational robustness
Data streams from environmental sensors and health sensors
Karine has been working with Yehia for a few years on the Polluscope project (ANR) and with Philippe as part of ACE-ICSEN, an IRS project of Paris-Saclay University. They met Cédric at the Center for Data Science and decided to raise the issue of Data Management, Data Analysis and Machine Learning together on IoT data.
On the one hand, StreamOps will rely on a sample of Polluscope data collected by portable multi-sensor boxes. The objective of Polluscope is to analyze, in all dimensions, all pollution information to characterize the exposure of an individual to air pollution. The SteamOps project will help make analysis and machine learning on these data streams.
On the other hand, a connected physiological multi-signal sensor (patch) is in parallel about to be tested by Philippe and Marc on pre-operative and especially post-operative medical follow-up. The idea is to stick on the thorax of the operated patient a multi-sensor patch to follow it remotely and permanently during the days following the intervention, in order to anticipate the risks of complications and to trigger the alert to good with a smart decision support system, without having to block the patient in a specialized medical unit.
The goal in StreamOps is to start from these two types of data and then create a generic application.
Being at the crossroads
Karine says: "We are going to develop new algorithms that will interface between a community that sees data as time series and analyzes it from a historical point of view, and another that sees IoT as a stream of data and analyzes all of these data dynamically, as they are recorded." The goal of StreamOps is to develop methods and algorithms to consider temporal order in data streams.
Cédric regularly works with industrials who are also interested in the possibility of having automatic tools to process data that comes in flow.
"It is not a question of proposing one more platform, explains Karine, but an integrating platform." The StreamOps team plans to collaborate with Albert Bifet, Telecom ParisTech, who developed the Massive Online Analysis (MOA) platform to create a compatible platform and achieve synergies.